AI 프렌즈 9회 학술 세미나
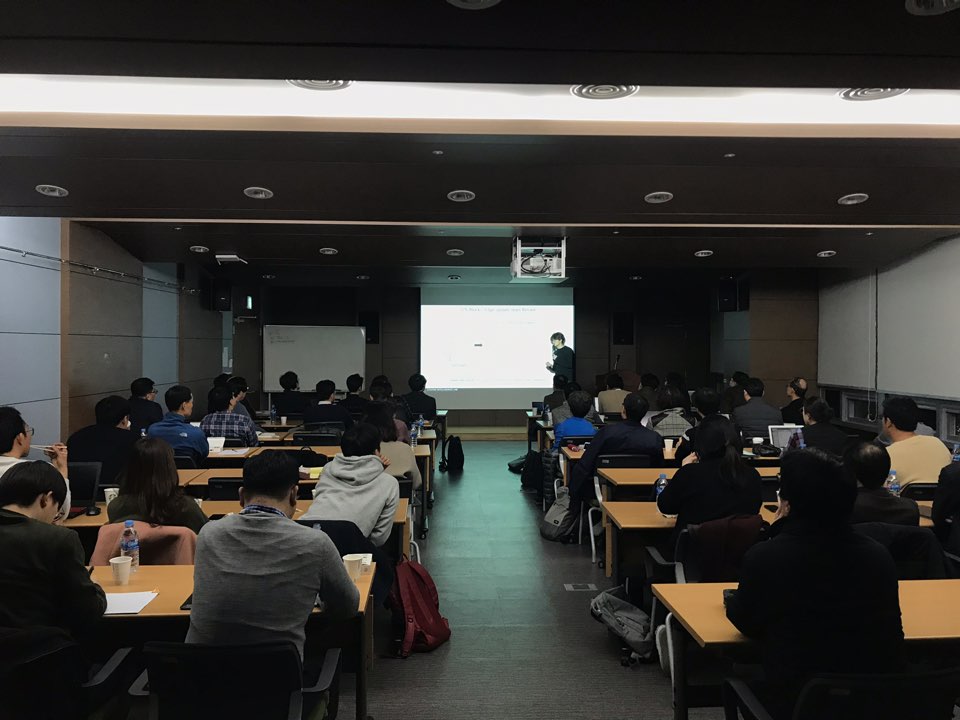
장소: 대덕테크비즈센터(TBC) 4층 대회의실 (412호)
일시: 2019년 3월 6일 저녁 7시~9시
발표 주제 및 발표자
1. Physics Induced Graph Neural Network (PGNN) for estimating wind farm power generations (박준영/KAIST 산업공학과 박사과정)
Precise and computationally efficient estimating power generation in a wind-farm is essential for the various purpose. For instance, estimating power generation profiles under external factors, such as the distribution of wind speed and wind direction, is required for the optimal wind turbine placement task. The aim of the study is suggesting a framework for estimating wind power generation in turbine levels under the variation of wind speed, wind direction, and wind farm layouts including the changing number of turbine cases. We achieved precise, computationally tractable, and differentiable wind farm power-estimating model by incorporating graph representations of wind farms and Physics induced Graph Neural Network (PGNN). We employed a directed graph for representing wind farm as a graph. In the graph, the nodes indicate the turbines in the farm and the edges denote the physical relationship between two turbines such as the Euclidean distances and contained angles. GWN learned the stationary wake patterns among the wind turbines by considering connecting structures among the nodes and the edges in the computational procedures of PGNN. We showed that combining the physical knowledge embedded in the graph formulation and data-driven approaches presented the generalization capability to PGNN. As a result, PGNN showed averagely <4% RMSE errors in the presence of wind speed, wind direction, and even farm layout variations. Moreover, we concluded that PGNN learned the proper wake interaction patterns among the wind turbines by showing the empirical estimation performance of PGNN in the scenario of the growing number of turbines.
2. RobustSTL: A Robust Seasonal-Trend Decomposition Algorithm for Long Time Series (AAAI 2019) (이도엽/POSTECH 박사과정)
최근에 발표된 논문으로 시계열 데이터의 예측, 이상탐지등을 위한 trend & seasonality 특성을 추출하는데 탁월한 성능을 보여주는 기법에 대한 이론 설명 및 코드 구현이 있을 예정입니다.
후원: 연구개발특구진흥재단, 대덕넷
문의: ai.friends.seminar@gmail.com,
Ai프렌즈 카카오톡 오픈 채팅방,
페이스북 홈페이지